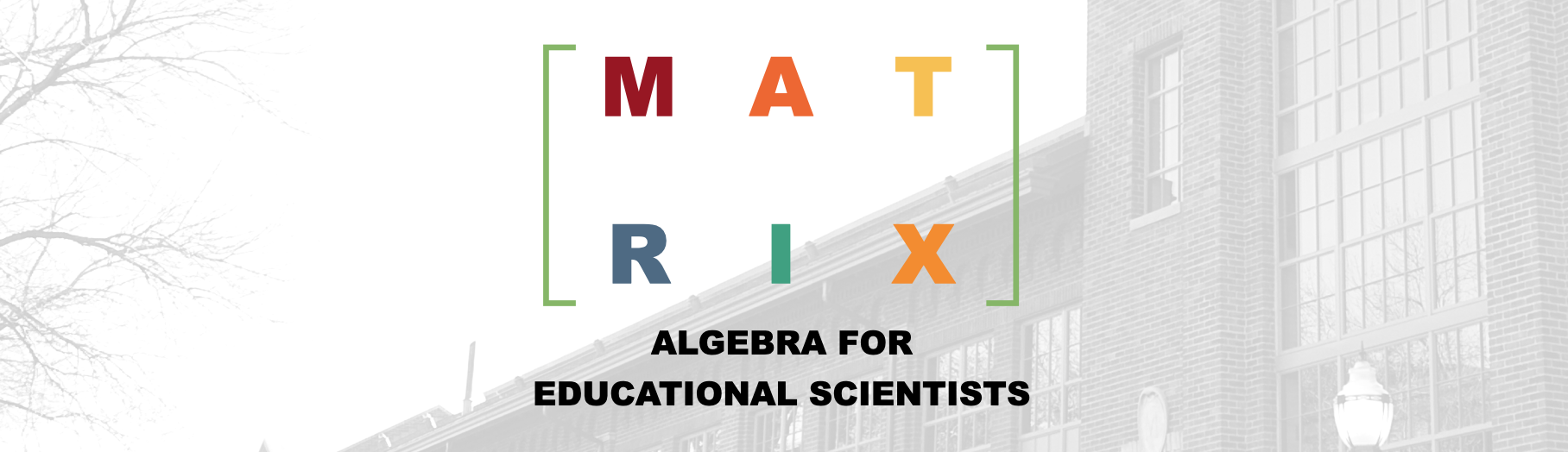
Chapter 23 Cholesky Decompostion
Another decomposition method is Cholesky (or Choleski) decomposition. The Cholesky decomposition method—used in statistical applications from nonlinear optimization, to Monte Carlo simulation methods, to Kalman filtering—is much more computationally efficient than the LU method. The Cholesky method decomposes a symmetric, positive definite matrix A into the product of two matrices, \(\mathbf{L}\) (a lower-triangular matrix) and \(\mathbf{L}^*\) (the conjugate transpose of \(\mathbf{L}\)).11
\[ \underset{n \times n}{\mathbf{A}} = \mathbf{LL}^* \]
The conjugate transpose is computed by taking the transpose of a matrix and then finding the complex conjugate of each element in the matrix. To understand what a complex conjugate is, we first remind you of the idea of a complex number. Remember that all real and imaginary numbers are complex numbers which can be expressed as \(a+bi\), where a is the real part of the number and b is the imaginary part of the number, and i is the square root of \(-1\). For example the number 2 can be expressed as \(2 + 0i\). Note: The complex conjugate of a real number is just the real number, since \(a+0i = a-0i=a\).
The complex conjugate of a number is itself a complex number that has the exact same real part and an imaginary part equal in magnitude but opposite in sign. For example the complex conjugate for the number \(3 + 2i\) is \(3 - 2i\).
As an example, say that matrix A was
\[ \mathbf{A} = \begin{bmatrix} 1 & 3+i & -2+3i \\ 0 & 4 & 0-i \\ \end{bmatrix} \]
The conjugate transpose of A, symbolized as \(\mathbf{A}^*\), can be found by first computing the transpose of A, and then finding the complex conjugate of each element in the transpose.
\[ \mathbf{A}^{\intercal} = \begin{bmatrix} 1 & 0 \\ 3+i0 & 4 \\ -2+3i & 0-i \end{bmatrix} \]
And,
\[ \mathbf{A}^{*} = \begin{bmatrix} 1 & 0 \\ 3-i0 & 4 \\ -2-3i & 0+i \end{bmatrix} \]
Many texts just present the notation for the Cholesky decomposition as \(\mathbf{A}=\mathbf{LL}^\intercal\), rather than \(\mathbf{A}=\mathbf{LL}^*\). This is because they make the further assumption that all the elements in A are real numbers. In that case, \(\mathbf{L}^*=\mathbf{L}^\intercal\). We will make that assumption and use that notation moving forward.
23.1 Example of Cholesky Decomposition
Say we wanted to compute a Cholsky decomposition on a \(2 \times 2\) symmetric matrix:
\[ \underset{2\times 2}{\mathbf{A}} = \begin{bmatrix} 5 & -4 \\ -4 & 5 \\ \end{bmatrix} \]
The Cholesky decomposition would factor A into the following:
\[ \begin{split} \underset{2\times 2}{\mathbf{A}} &= \underset{2\times 2}{\mathbf{L}}~\underset{2\times 2}{\mathbf{L}^\intercal}\\[1em] \begin{bmatrix} 5 & -4 \\ -4 & 5 \\ \end{bmatrix} &= \begin{bmatrix} l_{11} & 0 \\ l_{21} & l_{22} \\ \end{bmatrix} \begin{bmatrix} l_{11} & l_{21} \\ 0 & l_{22} \\ \end{bmatrix} \end{split} \]
Carrying out the matrix algebra we have the following three unique equations:
\[ \begin{split} 5 &= l_{11}(l_{11}) \\[0.5em] -4 &= l_{11}(l_{21}) \\[0.5em] 5 &= l_{21}(l_{21}) + l_{22}(l_{22}) \end{split} \]
Since we have three equations with three unknowns we can solve for each element in L.
\[ \begin{split} l_{11} &= \sqrt{5} \approx 2.24\\[0.5em] l_{21} &= \dfrac{-4}{\sqrt{5}} \approx -1.79 \\[0.5em] l_{22} &= \sqrt{1.8} \approx 1.34 \end{split} \]
So the Cholsky decomposition is,
\[ \begin{bmatrix} 5 & -4 \\ -4 & 5 \\ \end{bmatrix} = \begin{bmatrix} \sqrt{5} & 0 \\ \dfrac{-4}{\sqrt{5}} & \sqrt{1.8} \\ \end{bmatrix} \begin{bmatrix} \sqrt{5} & \dfrac{-4}{\sqrt{5}} \\ 0 & \sqrt{1.8} \\ \end{bmatrix} \]
Or using the approximations,
\[ \begin{bmatrix} 5 & -4 \\ -4 & 5 \\ \end{bmatrix} \approx \begin{bmatrix} 2.24 & 0 \\ -1.79 & 1.34 \\ \end{bmatrix} \begin{bmatrix} 2.24 & -1.79 \\ 0 & 1.34 \\ \end{bmatrix} \]
23.2 Cholesky Decomposition using R
We can use the chol()
function to compute the Cholesky decomposition. For example to carry out the Cholesky decomposition on A form the previous section, we would use the following syntax:
# Create A
= matrix(
A data = c(5, -4, -4, 5),
nrow = 2
)
# Cholesky decomposition
= chol(A)
cholesky_decomp
# View results
cholesky_decomp
[,1] [,2]
[1,] 2.236 -1.789
[2,] 0.000 1.342
Note that the output from chol()
is actually the transpose matrix, \(\mathbf{L}^\intercal\). To obtain L, we need to take the transpose of this output. We can also verify that the decomposition worked.
# Obtain L
t(cholesky_decomp)
[,1] [,2]
[1,] 2.236 0.000
[2,] -1.789 1.342
# Check results L(L^T) = A
t(cholesky_decomp) %*% cholesky_decomp
[,1] [,2]
[1,] 5 -4
[2,] -4 5
🚧 CAUTION: The chol()
function does not check for symmetry. If you use the function to decompose a nonsymmetric matrix, the results will likely be meaningless.
For example, consider the following nonsymmetric matrix:
\[ \mathbf{A} = \begin{bmatrix}5 & 1\\ -4 & 2\end{bmatrix} \]
Computing the decomposition, we get:
# Create A
= matrix(
A data = c(5, -4, 1, 2),
nrow = 2
)
# Cholsky decomposition
chol(A)
[,1] [,2]
[1,] 2.236 0.4472
[2,] 0.000 1.3416
Checking the matrix multiplication we do not get back to the original matrix, A:
# Check results
t(chol(A)) %*% chol(A)
[,1] [,2]
[1,] 5 1
[2,] 1 2
23.3 Statistical Application: Estimating Regression Coefficents with Cholesky Decomposition
We can again use the the OLS solution to compute the elements of b by solving the system of equations represented in:
\[ (\mathbf{X}^{\intercal}\mathbf{X})\mathbf{b} = \mathbf{X}^{\intercal}\mathbf{y} \]
So long as \(\mathbf{X}^\intercal\mathbf{X}\) is positive definite, we can use Cholesky decomposition to solve for the elements of b using the same two-step process we did for LU decomposition. Namely,
\[ \begin{split} &\mathrm{Solve~~}\mathbf{LZ} &= \mathbf{X}^{\intercal}\mathbf{y}~~\mathrm{for~}\mathbf{Z} \\[2ex] &\mathrm{Solve~~}\mathbf{L}^\intercal\mathbf{b} & =\mathbf{Z}~~\mathrm{for~}\mathbf{b} \end{split} \]
Using the same simulated data as the example from last chapter, consider the following simulated data set of \(n=50\) cases:
# Number of cases
= 50
n
# Create 50 x-values evenly spread b/w 1 and 500
= seq(from = 1, to = 500, len = n)
x
# Create X matrix
= cbind(1, x, x^2, x^3)
X
# Create b matrix
= matrix(
b data = c(1, 1, 1, 1),
nrow = 4
)
# Create vector of y-values
set.seed(1)
= X %*% b + rnorm(n, mean = 0, sd = 1) y
We can again use forwardsolve()
and backsolve()
to help solve the two equations.
# Obtain L^T and L
= chol(t(X)%*%X)
L_T = t(L_T)
L
# Solve for Z
= forwardsolve(L, crossprod(X,y))
Z
# Solve for b
= backsolve(L_T, Z)
b
# View b
b
[,1]
[1,] 0.9038
[2,] 1.0066
[3,] 1.0000
[4,] 1.0000
There are also variations on the Cholesky decomposition method, including LDL and LDL\(^\intercal\) decomposition. In these methods, D is a diagonal matrix.↩︎