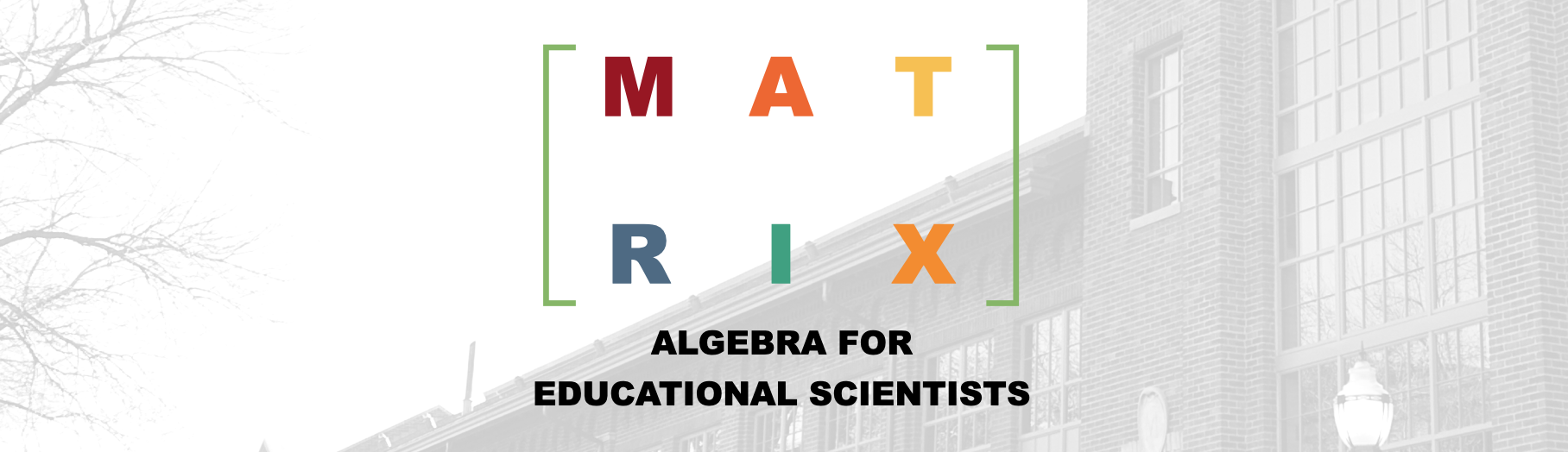
Chapter 26 Singular Value Decompostion
Singular value decomposition (SVD) is commonly used for data compression or variable reduction, and plays a large role in machine learning applications. SVD decomposes a matrix into the product of three matrices:
\[ \underset{m\times n}{\mathbf{A}} = \underset{m\times n}{\mathbf{U}}~ \underset{n\times n}{\mathbf{D}}~ \underset{n\times n}{\mathbf{V}^{\intercal}} \]
where, U and V are an orthogonal matrices, and D is a diagonal matrix. From the properties of the transpose, we know that,
\[ \mathbf{A}^{\intercal} = \mathbf{V}\mathbf{D}^{\intercal}\mathbf{U}^{\intercal} \]
And for mathematical convenience, we can take advantage that U and V are an orthogonal matrices (\(\mathbf{U}^{\intercal}\mathbf{U}=\mathbf{V}^{\intercal}\mathbf{V}=\mathbf{I}\)) by expressing two equations:
\[ \begin{split} \mathbf{A}\mathbf{A}^{\intercal}\mathbf{U} &= \mathbf{U}\mathbf{S}\mathbf{V}^{\intercal}\mathbf{V}\mathbf{D}^{\intercal}\mathbf{U}^{\intercal}\mathbf{U} \\[0.5em] &= \mathbf{U}\mathbf{D}^2 \end{split} \]
And,
\[ \begin{split} \mathbf{A}^{\intercal}\mathbf{A}\mathbf{V} &= \mathbf{V}\mathbf{D}^{\intercal}\mathbf{U}^{\intercal}\mathbf{U}\mathbf{D}\mathbf{V}^{\intercal}\mathbf{V}\\[0.5em] &= \mathbf{V}\mathbf{D}^2 \end{split} \]
These two equations are called eigenvalue equations, which show up all over the place in statistical work. These are easy to solve by computation. For example, we would solve the second eigenvalue equation to find V and D, and then find U by solving \(\mathbf{A}=\mathbf{UDV}^\intercal\) for U, namely:
\[ \mathbf{U} = \mathbf{A}\mathbf{V}\mathbf{D}^{-1} \]
26.1 SVD Using R
In practice, we will use the svd()
function in R to carry out singular value decomposition.
# Create A
= matrix(
A data = c(5, -4, 1, 2),
nrow = 2
)
# Singular value decomposition
= svd(A)
sv_decomp
# View results
sv_decomp
$d
[1] 6.422 2.180
$u
[,1] [,2]
[1,] -0.7630 0.6464
[2,] 0.6464 0.7630
$v
[,1] [,2]
[1,] -0.99659 0.08248
[2,] 0.08248 0.99659
The resulting decomposition is:
\[
\begin{split}
\mathbf{U} &= \begin{bmatrix}
-0.76 & 0.65 \\
0.65 & 0.76 \\
\end{bmatrix} \\[1em]
\mathbf{D} &= \begin{bmatrix}
6.42 & 0 \\
0 & 2.18 \\
\end{bmatrix} \\[1em]
\mathbf{V} &= \begin{bmatrix}
-1.00 & 0.08 \\
0.08 & 1.00 \\
\end{bmatrix}
\end{split}
\]
We can verify that \(\mathbf{A}=\mathbf{USV}^\intercal\). Note that the svd()
function outputs the diagonal elements of the D matrix, so we need to use the diag()
function to create the actual D matrix.
# Verify the results of the SVD
$u %*% diag(sv_decomp$d) %*% t(sv_decomp$v) sv_decomp
[,1] [,2]
[1,] 5 1
[2,] -4 2