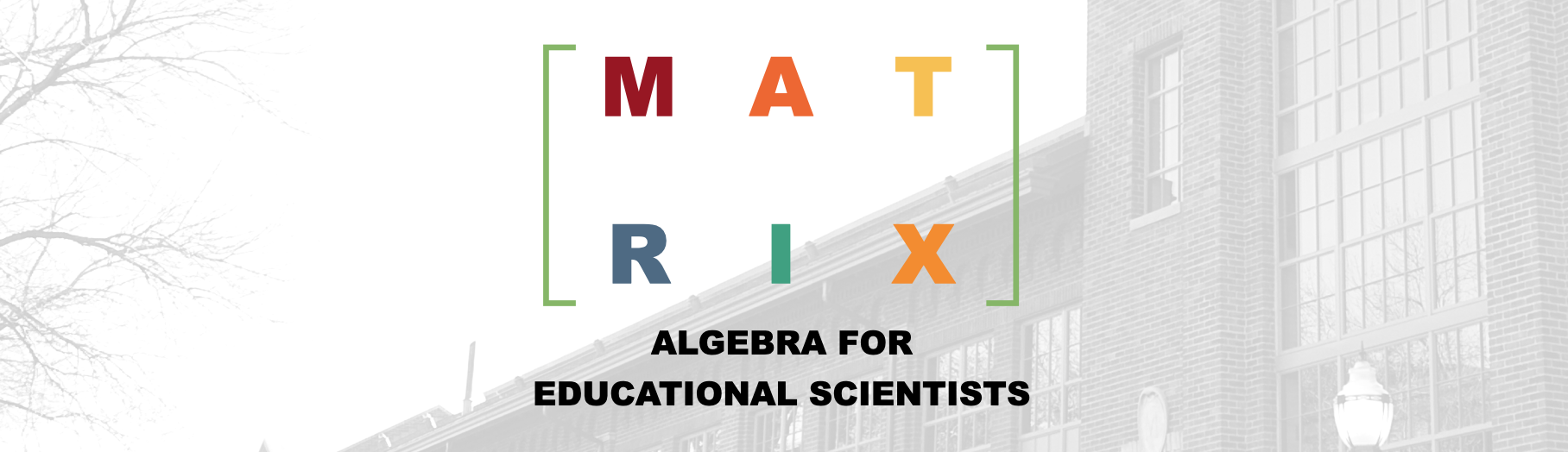
Chapter 24 QR Decompostion
Another decomposition method is QR decomposition. One advantage of QR decomposition over LU decomposition is that this method does not require that the decomposition be carried out on a square matrix. QR decomposition results in factoring matrix A (having independent columns) into the product of two matrices, namely Q and R: .
\[ \underset{m\times n}{\mathbf{A}} = \underset{m\times m}{\mathbf{Q}}~ \underset{m\times n}{\mathbf{R}} \]
where Q is an orthogonal matrix12 and R is an upper-triangular matrix.
24.1 QR Decomposition using R
Although there is a way to hand-calculate the matrices Q and R (e.g., using the Gram-Schmidt process), we will rely on computation. To carry out a QR decomposition in R, we will use the qr()
function which factors the matrix and returns a list of output related to the QR decomposition. To extract the actual Q and R matrices from this output, we will use the qr.Q()
and qr.R()
functions, respectively.
# Create matrix A
= matrix(
A data = c(5, -4, 1, 2),
nrow = 2
)
# Carry out QR decomposition
= qr(A)
qr_decomp
# View Q matrix
qr.Q(qr_decomp)
[,1] [,2]
[1,] -0.7809 0.6247
[2,] 0.6247 0.7809
# View R matrix
qr.R(qr_decomp)
[,1] [,2]
[1,] -6.403 0.4685
[2,] 0.000 2.1864
We find:
\[ \begin{split} \mathbf{A} &= \mathbf{QR} \\[2ex] \begin{bmatrix}5 & 1 \\ -4 & 2\end{bmatrix} &= \begin{bmatrix}-0.7809 & 0.6247 \\ 0.6247 & 0.7809\end{bmatrix}\begin{bmatrix}-6.403 & 0.4685 \\ 0 & 2.1864\end{bmatrix} \end{split} \]
You can see that R is an upper-triangular matrix, and we can check that Q is orthonormal by testing whether \(\mathbf{Q}^{\intercal} = \mathbf{Q}^{-1}\).
# Q^T
t(qr.Q(qr_decomp))
[,1] [,2]
[1,] -0.7809 0.6247
[2,] 0.6247 0.7809
# Q^-1
solve(qr.Q(qr_decomp))
[,1] [,2]
[1,] -0.7809 0.6247
[2,] 0.6247 0.7809
We can also check to see that the product of the decomposed matrices is A.
# A = QR?
qr.Q(qr_decomp) %*% qr.R(qr_decomp)
[,1] [,2]
[1,] 5 1
[2,] -4 2
24.2 Statistical Application: Estimating Regression Coefficents with QR Decomposition
We can use the two decomposed matrices, Q and R, to compute the elements of b in the OLS solution, solving the system of equations represented in:
\[ (\mathbf{X}^{\intercal}\mathbf{X})\mathbf{b} = \mathbf{X}^{\intercal}\mathbf{y} \]
Since QR decomposition works on non-square matrices, we can decompose X as:
\[ \mathbf{X} = \mathbf{QR} \]
Then the OLS equation can be written as:
\[ \begin{split} (\mathbf{QR})^{\intercal}\mathbf{QR}\mathbf{b} &= (\mathbf{QR})^{\intercal}\mathbf{y} \\[2ex] \mathbf{R}^{\intercal}\mathbf{Q}^{\intercal}\mathbf{QR}\mathbf{b} &= \mathbf{R}^{\intercal}\mathbf{Q}^{\intercal}\mathbf{y} \end{split} \]
Since Q is orthonormal, and \(\mathbf{QQ}^\intercal = \mathbf{Q}^\intercal\mathbf{Q}=\mathbf{I}\), then this reduces to:
\[ \begin{split} \mathbf{R}^{\intercal}\mathbf{I}\mathbf{R}\mathbf{b} &= \mathbf{R}^{\intercal}\mathbf{Q}^{\intercal}\mathbf{y} \\[2ex] \mathbf{R}^{\intercal}\mathbf{R}\mathbf{b} &= \mathbf{R}^{\intercal}\mathbf{Q}^{\intercal}\mathbf{y} \\[2ex] \mathbf{R}\mathbf{b} &= \mathbf{Q}^{\intercal}\mathbf{y} \end{split} \]
Since R is an upper-triangular matrix, we can use back substitution to solve for the elements of b. Using the same simulated data as the example from last chapter, consider the following simulated data set of \(n=50\) cases:
# Number of cases
= 50
n
# Create 50 x-values evenly spread b/w 1 and 500
= seq(from = 1, to = 500, len = n)
x
# Create X matrix
= cbind(1, x, x^2, x^3)
X colnames(X) <- c("Intercept", "x", "x2", "x3")
# Create b matrix
= matrix(
b data = c(1, 1, 1, 1),
nrow = 4
)
# Create vector of y-values
set.seed(1)
= X %*% b + rnorm(n, mean = 0, sd = 1) y
We can use QR decomposition to solve for b.
# Carry out QR decomposition
= qr(X)
QR
# Solve for b
backsolve(qr.R(QR), t(qr.Q(QR)) %*% y)
[,1]
[1,] 0.9038
[2,] 1.0066
[3,] 1.0000
[4,] 1.0000
QR decomposition is how the lm()
function computes the regression coefficients.
The columns of an orthogonal matrix are orthogonal unit vectors and it has the property that \(\mathbf{Q}^{T}\mathbf{Q}=\mathbf{I}\) or that \(\mathbf{Q}^{\intercal} = \mathbf{Q}^{-1}\).↩︎