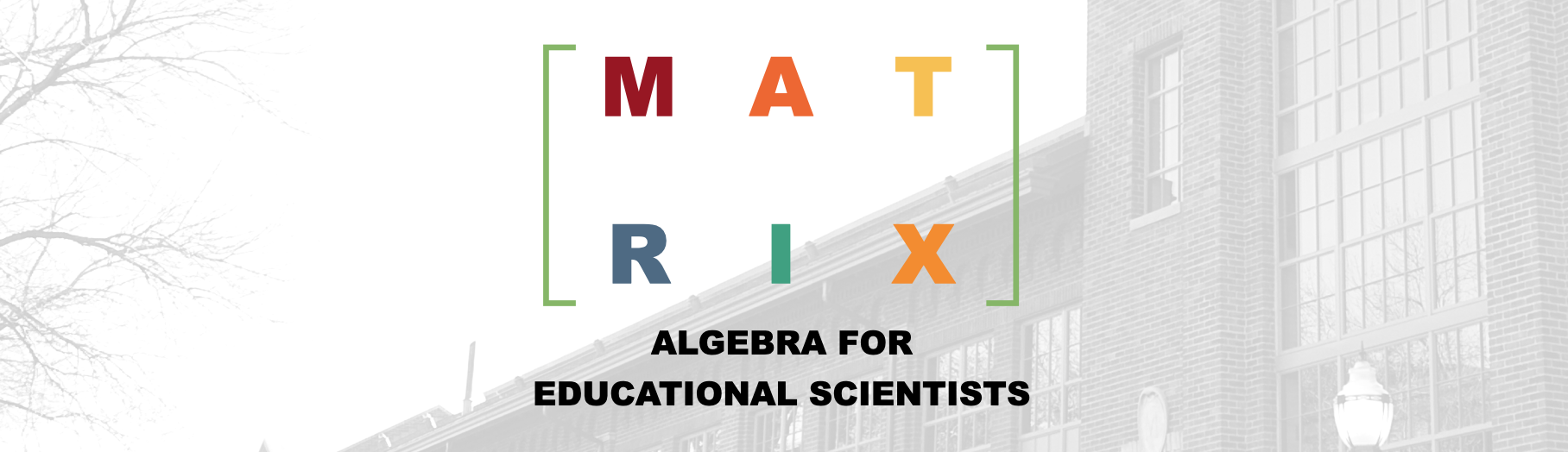
Chapter 15 Statistical Application: SSCP, Variance–Covariance, and Correlation Matrices
In this chapter, we will provide an example of how matrix operations are used in statistics to compute a sum of squares and cross-product (SSCP) matrix. To do this we will consider the following data set:
ID | SAT | GPA | Self-Esteem | IQ |
---|---|---|---|---|
1 | 560 | 3.0 | 11 | 112 |
2 | 780 | 3.9 | 10 | 143 |
3 | 620 | 2.9 | 19 | 124 |
4 | 600 | 2.7 | 7 | 129 |
5 | 720 | 3.7 | 18 | 130 |
6 | 380 | 2.4 | 13 | 82 |
The data matrix (omitting ID values) could be conceived of as a \(6 \times 4\) matrix:
\[ \mathbf{X} = \begin{bmatrix} 560 & 3.0 & 11 & 112 \\ 780 & 3.9 & 10 & 143 \\ 620 & 2.9 & 19 & 124 \\ 600 & 2.7 & 7 & 129 \\ 720 & 3.7 & 18 & 130 \\ 380 & 2.4 & 13 & 82 \end{bmatrix} \]
15.1 Deviation Scores
Since so much of statistics is dependent on the use of deviation scores, we will compute a matrix of means, where each column corresponds to the mean of the corresponding column mean of X, and subtract that from the original matrix X.
\[ \begin{split} \mathbf{D} &= \mathbf{X} - \mathbf{M} \\[2ex] &= \begin{bmatrix} 560 & 3.0 & 11 & 112 \\ 780 & 3.9 & 10 & 143 \\ 620 & 2.9 & 19 & 124 \\ 600 & 2.7 & 7 & 129 \\ 720 & 3.7 & 18 & 130 \\ 380 & 2.4 & 13 & 82 \end{bmatrix} - \begin{bmatrix} 610 & 3.1 & 13 & 120 \\ 610 & 3.1 & 13 & 120 \\ 610 & 3.1 & 13 & 120 \\ 610 & 3.1 & 13 & 120 \\ 610 & 3.1 & 13 & 120 \\ 610 & 3.1 & 13 & 120 \end{bmatrix} \\[2ex] &= \begin{bmatrix} -50 & -0.1 & -2 & -8 \\ 170 & 0.8 & -3 & 23 \\ 10 & -0.2 & 6 & 4 \\ -10 & -0.4 & -6 & 9 \\ 110 & 0.6 & 5 & 10 \\ -230 & -0.7 & 0 & -38 \end{bmatrix} \end{split} \]
Using R, the following commands produce the deviation matrix.
# Create X
= matrix(
X data = c(560, 3.0, 11, 112,
780, 3.9, 10, 143,
620, 2.9, 19, 124,
600, 2.7, 7, 129,
720, 3.7, 18, 130,
380, 2.4, 13, 82),
byrow = TRUE,
ncol = 4
)
# Create a ones column vector with six elements
= rep(1, 6)
ones
# Compute M (mean matrix)
= ones %*% t(ones) %*% X * (1/6)
M M
[,1] [,2] [,3] [,4]
[1,] 610 3.1 13 120
[2,] 610 3.1 13 120
[3,] 610 3.1 13 120
[4,] 610 3.1 13 120
[5,] 610 3.1 13 120
[6,] 610 3.1 13 120
# Compute deviation matrix (D)
= X - M
D D
[,1] [,2] [,3] [,4]
[1,] -50 -0.1 -2 -8
[2,] 170 0.8 -3 23
[3,] 10 -0.2 6 4
[4,] -10 -0.4 -6 9
[5,] 110 0.6 5 10
[6,] -230 -0.7 0 -38
15.1.1 Creating the Mean Matrix
Here we used matrix operations to compute the mean matrix rather than built-in R functions. Taking a ones column vector of n elements and post-multiplying by its transpose produces a \(n \times n\) matrix where all the elements are one; in our case we get a \(6 \times 6\) ones matrix.
%*% t(ones) ones
[,1] [,2] [,3] [,4] [,5] [,6]
[1,] 1 1 1 1 1 1
[2,] 1 1 1 1 1 1
[3,] 1 1 1 1 1 1
[4,] 1 1 1 1 1 1
[5,] 1 1 1 1 1 1
[6,] 1 1 1 1 1 1
This ones matrix is then post-multiplied by X. Since we are post-multiplyinga ones matrix, the resultin matrix will have the same elements in every row. Moreover these elements will be the column sums of X.
%*% t(ones) %*% X ones
[,1] [,2] [,3] [,4]
[1,] 3660 18.6 78 720
[2,] 3660 18.6 78 720
[3,] 3660 18.6 78 720
[4,] 3660 18.6 78 720
[5,] 3660 18.6 78 720
[6,] 3660 18.6 78 720
# Compute column sums
colSums(X)
[1] 3660.0 18.6 78.0 720.0
Finally, since we want the means, we multiply by the scalar \(\frac{1}{n}\), where n is the number of rows. This gets us the mean matrix, all via matrix operations.
%*% t(ones) %*% X * (1/6) ones
[,1] [,2] [,3] [,4]
[1,] 610 3.1 13 120
[2,] 610 3.1 13 120
[3,] 610 3.1 13 120
[4,] 610 3.1 13 120
[5,] 610 3.1 13 120
[6,] 610 3.1 13 120
Using mathematical notation, the deviation matrix can be expressed as:
\[ \begin{split} \underset{6 \times 4}{\mathbf{D}} &= \underset{6 \times 4}{\mathbf{X}} - \underset{6 \times 4}{\mathbf{M}} \\[2ex] &= \begin{bmatrix} X_{1_{1}} & X_{2_{1}} & X_{3_{1}} & X_{4_{1}} \\ X_{1_{2}} & X_{2_{2}} & X_{3_{2}} & X_{4_{2}} \\ X_{1_{3}} & X_{2_{3}} & X_{3_{3}} & X_{4_{3}} \\ X_{1_{4}} & X_{2_{4}} & X_{3_{4}} & X_{4_{4}} \\ X_{1_{5}} & X_{2_{5}} & X_{3_{5}} & X_{4_{5}} \\ X_{1_{6}} & X_{2_{6}} & X_{3_{6}} & X_{4_{6}} \end{bmatrix} - \begin{bmatrix} \bar{X}_1 & \bar{X}_2 & \bar{X}_3 & \bar{X}_4 \\ \bar{X}_1 & \bar{X}_2 & \bar{X}_3 & \bar{X}_4 \\ \bar{X}_1 & \bar{X}_2 & \bar{X}_3 & \bar{X}_4 \\ \bar{X}_1 & \bar{X}_2 & \bar{X}_3 & \bar{X}_4 \\ \bar{X}_1 & \bar{X}_2 & \bar{X}_3 & \bar{X}_4 \\ \bar{X}_1 & \bar{X}_2 & \bar{X}_3 & \bar{X}_4 \end{bmatrix} \\[2ex] &= \begin{bmatrix} (X_{1_{1}} - \bar{X}_1) & (X_{2_{1}} - \bar{X}_2) & (X_{3_{1}} - \bar{X}_3) & (X_{4_{1}} - \bar{X}_4) \\ (X_{1_{2}} - \bar{X}_1) & (X_{2_{2}} - \bar{X}_2) & (X_{3_{2}} - \bar{X}_3) & (X_{4_{2}} - \bar{X}_4) \\ (X_{1_{3}} - \bar{X}_1) & (X_{2_{3}} - \bar{X}_2) & (X_{3_{3}} - \bar{X}_3) & (X_{4_{3}} - \bar{X}_4) \\ (X_{1_{4}} - \bar{X}_1) & (X_{2_{4}} - \bar{X}_2) & (X_{3_{4}} - \bar{X}_3) & (X_{4_{4}} - \bar{X}_4) \\ (X_{1_{5}} - \bar{X}_1) & (X_{2_{5}} - \bar{X}_2) & (X_{3_{5}} - \bar{X}_3) & (X_{4_{5}} - \bar{X}_4) \\ (X_{1_{6}} - \bar{X}_1) & (X_{2_{6}} - \bar{X}_2) & (X_{3_{6}} - \bar{X}_3) & (X_{4_{6}} - \bar{X}_4) \end{bmatrix} \end{split} \]
15.2 SSCP Matrix
To obtain the sums of squares and cross products (SSCP) matrix, we can pre-multiply D by its transpose.
\[ \begin{split} \underset{4 \times 4}{\mathbf{SSCP}} &= \underset{4 \times 6}{\mathbf{D}}^\intercal\underset{6 \times 4}{\mathbf{D}} \\[2ex] &= \begin{bmatrix} (X_{1_{1}} - \bar{X}_1) & (X_{1_{2}} - \bar{X}_1) & (X_{1_{3}} - \bar{X}_1) & (X_{1_{4}} - \bar{X}_1) & (X_{1_{5}} - \bar{X}_1) & (X_{1_{6}} - \bar{X}_1) \\ (X_{2_{1}} - \bar{X}_2) & (X_{2_{2}} - \bar{X}_2) & (X_{2_{3}} - \bar{X}_2) & (X_{2_{4}} - \bar{X}_2) & (X_{2_{5}} - \bar{X}_2) & (X_{2_{6}} - \bar{X}_2) \\ (X_{3_{1}} - \bar{X}_3) & (X_{3_{2}} - \bar{X}_3) & (X_{3_{3}} - \bar{X}_3) & (X_{3_{4}} - \bar{X}_3) & (X_{3_{5}} - \bar{X}_3) & (X_{3_{6}} - \bar{X}_3) \\ (X_{4_{1}} - \bar{X}_4) & (X_{4_{2}} - \bar{X}_4) & (X_{4_{3}} - \bar{X}_4) & (X_{4_{4}} - \bar{X}_4) & (X_{4_{5}} - \bar{X}_4) & (X_{4_{6}} - \bar{X}_4) \end{bmatrix} \begin{bmatrix} (X_{1_{1}} - \bar{X}_1) & (X_{2_{1}} - \bar{X}_2) & (X_{3_{1}} - \bar{X}_3) & (X_{4_{1}} - \bar{X}_4) \\ (X_{1_{2}} - \bar{X}_1) & (X_{2_{2}} - \bar{X}_2) & (X_{3_{2}} - \bar{X}_3) & (X_{4_{2}} - \bar{X}_4) \\ (X_{1_{3}} - \bar{X}_1) & (X_{2_{3}} - \bar{X}_2) & (X_{3_{3}} - \bar{X}_3) & (X_{4_{3}} - \bar{X}_4) \\ (X_{1_{4}} - \bar{X}_1) & (X_{2_{4}} - \bar{X}_2) & (X_{3_{4}} - \bar{X}_3) & (X_{4_{4}} - \bar{X}_4) \\ (X_{1_{5}} - \bar{X}_1) & (X_{2_{5}} - \bar{X}_2) & (X_{3_{5}} - \bar{X}_3) & (X_{4_{5}} - \bar{X}_4) \\ (X_{1_{6}} - \bar{X}_1) & (X_{2_{6}} - \bar{X}_2) & (X_{3_{6}} - \bar{X}_3) & (X_{4_{6}} - \bar{X}_4) \end{bmatrix} \\[2ex] &= \begin{bmatrix} \sum (X_{1_{i}} - \bar{X}_1)^2 & \sum (X_{1_{i}} - \bar{X}_1)(X_{2_{i}} - \bar{X}_2) & \sum (X_{1_{i}} - \bar{X}_1)(X_{3_{i}} - \bar{X}_3) & \sum (X_{1_{i}} - \bar{X}_1)(X_{4_{i}} - \bar{X}_4) \\ \sum (X_{1_{i}} - \bar{X}_1)(X_{2_{i}} - \bar{X}_2) & \sum (X_{2_{i}} - \bar{X}_2)^2 & \sum (X_{2_{i}} - \bar{X}_2)(X_{3_{i}} - \bar{X}_3) & \sum (X_{2_{i}} - \bar{X}_2)(X_{4_{i}} - \bar{X}_4) \\ \sum (X_{1_{i}} - \bar{X}_1)(X_{3_{i}} - \bar{X}_3) & \sum (X_{2_{i}} - \bar{X}_2)(X_{3_{i}} - \bar{X}_3) & \sum (X_{3_{i}} - \bar{X}_3)^2 & \sum (X_{3_{i}} - \bar{X}_3)(X_{4_{i}} - \bar{X}_4) \\ \sum (X_{1_{i}} - \bar{X}_1)(X_{4_{i}} - \bar{X}_4) & \sum (X_{2_{i}} - \bar{X}_2)(X_{4_{i}} - \bar{X}_4) & \sum (X_{3_{i}} - \bar{X}_3)(X_{4_{i}} - \bar{X}_4) & \sum (X_{4_{i}} - \bar{X}_4)^2 \end{bmatrix} \end{split} \]
The diagonal of the SSCP matrix contains the sums of squared deviations for each of the variables (columns) represented in X. For example, the element in the first row and first column of the SSCP matrix (\(\mathbf{SSCP}_{11}\)) is the sum of squared deviations for the SAT variable. The off-diagonal elements in the SSCP matrix are the cross-products of the deviation scores between the different variables. That is:
\[ \mathbf{SSCP} = \begin{bmatrix} \mathrm{SS}_1 & \mathrm{CP}_{12} & \mathrm{CP}_{13} & \mathrm{CP}_{14} \\ \mathrm{CP}_{21} & \mathrm{SS}_2 & \mathrm{CP}_{23} & \mathrm{CP}_{24} \\ \mathrm{CP}_{21} & \mathrm{CP}_{32} & \mathrm{SS}_3 & \mathrm{CP}_{34} \\ \mathrm{CP}_{41} & \mathrm{CP}_{42} & \mathrm{CP}_{43} & \mathrm{SS}_4 \end{bmatrix} \] Also note that the SSCP matrix is square and symmetric.
Using R:
# Compute SSCP matrix
= t(D) %*% D
SSCP
# View SSCP matrix
SSCP
[,1] [,2] [,3] [,4]
[1,] 96600 370.0 260 14100.0
[2,] 370 1.7 2 47.4
[3,] 260 2.0 110 -33.0
[4,] 14100 47.4 -33 2234.0
The SSCP matrix is a scalar multiple of the variance–covariance matrix (\(\boldsymbol{\Sigma}\)). Namely,
\[ \mathbf{SSCP} = n (\boldsymbol{\Sigma}) \]
That is if me multiply the SSCP matrix by \(\frac{1}{n}\) we obtain the variance–covariance matrix. Mathematically,
\[ \begin{split} \underset{4 \times 4}{\boldsymbol{\Sigma}} &= \frac{1}{n}(\underset{4 \times 4}{\mathbf{SSCP}}) \\[2ex] &= \frac{1}{n} \begin{bmatrix} \sum (X_{1_{i}} - \bar{X}_1)^2 & \sum (X_{1_{i}} - \bar{X}_1)(X_{2_{i}} - \bar{X}_2) & \sum (X_{1_{i}} - \bar{X}_1)(X_{3_{i}} - \bar{X}_3) & \sum (X_{1_{i}} - \bar{X}_1)(X_{4_{i}} - \bar{X}_4) \\ \sum (X_{1_{i}} - \bar{X}_1)(X_{2_{i}} - \bar{X}_2) & \sum (X_{2_{i}} - \bar{X}_2)^2 & \sum (X_{2_{i}} - \bar{X}_2)(X_{3_{i}} - \bar{X}_3) & \sum (X_{2_{i}} - \bar{X}_2)(X_{4_{i}} - \bar{X}_4) \\ \sum (X_{1_{i}} - \bar{X}_1)(X_{3_{i}} - \bar{X}_3) & \sum (X_{2_{i}} - \bar{X}_2)(X_{3_{i}} - \bar{X}_3) & \sum (X_{3_{i}} - \bar{X}_3)^2 & \sum (X_{3_{i}} - \bar{X}_3)(X_{4_{i}} - \bar{X}_4) \\ \sum (X_{1_{i}} - \bar{X}_1)(X_{4_{i}} - \bar{X}_4) & \sum (X_{2_{i}} - \bar{X}_2)(X_{4_{i}} - \bar{X}_4) & \sum (X_{3_{i}} - \bar{X}_3)(X_{4_{i}} - \bar{X}_4) & \sum (X_{4_{i}} - \bar{X}_4)^2 \end{bmatrix} \\[2ex] &= \begin{bmatrix} \frac{\sum (X_{1_{i}} - \bar{X}_1)^2}{n} & \frac{\sum (X_{1_{i}} - \bar{X}_1)(X_{2_{i}} - \bar{X}_2)}{n} & \frac{\sum (X_{1_{i}} - \bar{X}_1)(X_{3_{i}} - \bar{X}_3)}{n} & \frac{\sum (X_{1_{i}} - \bar{X}_1)(X_{4_{i}} - \bar{X}_4)}{n} \\ \frac{\sum (X_{1_{i}} - \bar{X}_1)(X_{2_{i}} - \bar{X}_2)}{n} & \frac{\sum (X_{2_{i}} - \bar{X}_2)^2}{n} & \frac{\sum (X_{2_{i}} - \bar{X}_2)(X_{3_{i}} - \bar{X}_3)}{n} & \frac{\sum (X_{2_{i}} - \bar{X}_2)(X_{4_{i}} - \bar{X}_4)}{n} \\ \frac{\sum (X_{1_{i}} - \bar{X}_1)(X_{3_{i}} - \bar{X}_3)}{n} & \frac{\sum (X_{2_{i}} - \bar{X}_2)(X_{3_{i}} - \bar{X}_3)}{n} & \frac{\sum (X_{3_{i}} - \bar{X}_3)^2}{n} & \frac{\sum (X_{3_{i}} - \bar{X}_3)(X_{4_{i}} - \bar{X}_4)}{n} \\ \frac{\sum (X_{1_{i}} - \bar{X}_1)(X_{4_{i}} - \bar{X}_4)}{n} & \frac{\sum (X_{2_{i}} - \bar{X}_2)(X_{4_{i}} - \bar{X}_4)}{n} & \frac{\sum (X_{3_{i}} - \bar{X}_3)(X_{4_{i}} - \bar{X}_4)}{n} & \frac{\sum (X_{4_{i}} - \bar{X}_4)^2}{n} \end{bmatrix} \end{split} \]
The diagonal of the \(\boldsymbol{\Sigma}\) matrix contains the variances for each of the variables (columns) represented in X. For example, the element in the first row and first column of \(\boldsymbol{\Sigma}\) (\(\boldsymbol{\Sigma}_{11}\)) is the variance for the SAT variable. The off-diagonal elements in \(\boldsymbol{\Sigma}\) are the covariances between the different variables. That is:
\[ \boldsymbol{\Sigma} = \begin{bmatrix} \mathrm{Var}(X_1) & \mathrm{Cov}(X_1,X_2) & \mathrm{Cov}(X_1,X_3) & \mathrm{Cov}(X_1,X_4) \\ \mathrm{Cov}(X_2,X_1) & \mathrm{Var}(X_2) & \mathrm{Cov}(X_2,X_3) & \mathrm{Cov}(X_2,X_4) \\ \mathrm{Cov}(X_3,X_1) & \mathrm{Cov}(X_3,X_2) & \mathrm{Var}(X_3) & \mathrm{Cov}(X_3,X_4) \\ \mathrm{Cov}(X_4,X_1) & \mathrm{Cov}(X_4,X_2) & \mathrm{Cov}(X_4,X_3) & \mathrm{Var}(X_4) \end{bmatrix} \]
Similar to the SSCP matrix, \(\boldsymbol{\Sigma}\) is also a square, symmetric matrix.
Using R:
# Compute SIGMA matrix
= SSCP * (1/6)
SIGMA
# View SIGMA matrix
SIGMA
[,1] [,2] [,3] [,4]
[1,] 16100.00000 61.6666667 43.3333333 2350.0000
[2,] 61.66667 0.2833333 0.3333333 7.9000
[3,] 43.33333 0.3333333 18.3333333 -5.5000
[4,] 2350.00000 7.9000000 -5.5000000 372.3333
The var()
function can also be applied to X directly to obtain the \(\boldsymbol{\Sigma}\) matrix. Note that the var()
function uses \(\frac{1}{n-1}\) rather than \(\frac{1}{n}\) as the scalar multiple to obtain the variance–covariance matrix.
# Obtain SIGMA directly
var(X)
[,1] [,2] [,3] [,4]
[1,] 19320 74.00 52.0 2820.00
[2,] 74 0.34 0.4 9.48
[3,] 52 0.40 22.0 -6.60
[4,] 2820 9.48 -6.6 446.80
# Check
* (1/5) SSCP
[,1] [,2] [,3] [,4]
[1,] 19320 74.00 52.0 2820.00
[2,] 74 0.34 0.4 9.48
[3,] 52 0.40 22.0 -6.60
[4,] 2820 9.48 -6.6 446.80
15.3 Correlation Matrix
We can convert \(\boldsymbol{\Sigma}\) to a correlation matrix by standardizing it; that is dividing each element by its corresponding standard deviation. This is equivalent to pre- and post-multiplying \(\boldsymbol{\Sigma}\) by a scaling matrix, S, a diagonal matrix with elements equal to the reciprocal of the standard deviations of each of the variables:
\[ \mathbf{S} = \begin{bmatrix} \frac{1}{\mathrm{SD}(X_1)} & 0 & 0 & 0 \\ 0 & \frac{1}{\mathrm{SD}(X_2)} & 0 & 0 \\ 0 & 0 & \frac{1}{\mathrm{SD}(X_3)} & 0 \\ 0 & 0 & 0 & \frac{1}{\mathrm{SD}(X_4)} \end{bmatrix} \]
Employing R, we can obtain the scaling matrix by first pulling out the diagonal elements of \(\boldsymbol{\Sigma}\) (the variances) and then using those to create S.
# Get variances
= diag(SIGMA)
V V
[1] 1.610000e+04 2.833333e-01 1.833333e+01 3.723333e+02
# Create S
= diag(1 / sqrt(V))
S S
[,1] [,2] [,3] [,4]
[1,] 0.007881104 0.000000 0.0000000 0.00000000
[2,] 0.000000000 1.878673 0.0000000 0.00000000
[3,] 0.000000000 0.000000 0.2335497 0.00000000
[4,] 0.000000000 0.000000 0.0000000 0.05182437
We can now pre- and post-multiply \(\boldsymbol{\Sigma}\) by S to obtain the correlation matrix, R. That is,
\[ \mathbf{R} = \mathbf{S}\boldsymbol{\Sigma}\mathbf{S} \] Since both S and \(\boldsymbol{\Sigma}\) are \(4 \times 4\) matrices, R will also be a \(4 \times 4\) matrix. Moreover, R will also be both square and symmetric.
= S %*% SIGMA %*% S
R R
[,1] [,2] [,3] [,4]
[1,] 1.00000000 0.9130377 0.07976061 0.95981817
[2,] 0.91303768 1.0000000 0.14625448 0.76915222
[3,] 0.07976061 0.1462545 1.00000000 -0.06656961
[4,] 0.95981817 0.7691522 -0.06656961 1.00000000
Again, the cor()
function could be applied directly to X, noting that the SSCP matrix would be scaled by \(\frac{1}{n-1}\).
15.4 Standardized Scores
Standardized scores (z-scores) can be computed for the original values in X by post-multiplying the deviation matrix (D) by the same scaling matrix, S.
\[ \mathbf{Z} = \mathbf{DS} \]
# Compute standardized scores
= D %*% S
Z Z
[,1] [,2] [,3] [,4]
[1,] -0.39405520 -0.1878673 -0.4670994 -0.4145950
[2,] 1.33978769 1.5029383 -0.7006490 1.1919605
[3,] 0.07881104 -0.3757346 1.4012981 0.2072975
[4,] -0.07881104 -0.7514691 -1.4012981 0.4664193
[5,] 0.86692145 1.1272037 1.1677484 0.5182437
[6,] -1.81265393 -1.3150710 0.0000000 -1.9693261
We can also use Z to compute the correlation matrix,
\[ \mathbf{R} = \frac{\mathbf{Z}^\intercal\mathbf{Z}}{n} \]
# Compute R
t(Z) %*% Z * (1/6)
[,1] [,2] [,3] [,4]
[1,] 1.00000000 0.9130377 0.07976061 0.95981817
[2,] 0.91303768 1.0000000 0.14625448 0.76915222
[3,] 0.07976061 0.1462545 1.00000000 -0.06656961
[4,] 0.95981817 0.7691522 -0.06656961 1.00000000