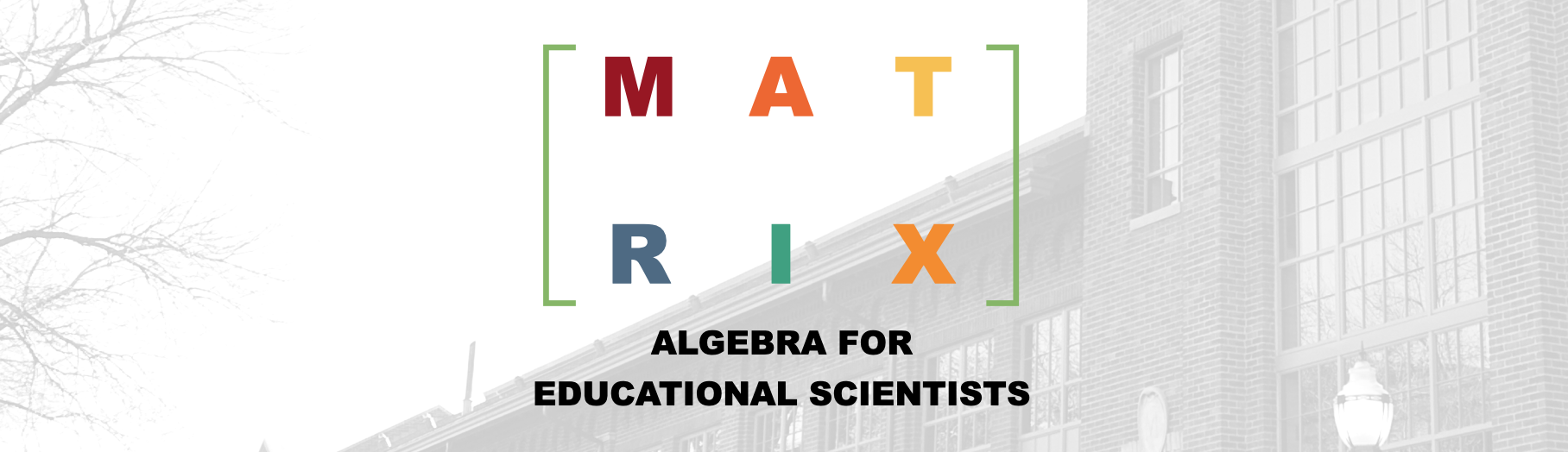
Chapter 21 LU Decompostion
One common method of matrix decomposition is LU decomposition. Commonly used to computationally solve systems of equations, and to find the determinant of a matrix, LU decomposition decomposes a square matrix into a pair of triangular matrices to more easily carry out Gaussian elimination. It is based on a theorem in linear algebra, which states:
Any nonsingular, square matrix, A, can be written as the product of two triangular matrices, L and U, of the same order such that matrix L is a lower-triangular matrix (all elements above the main diagonal are 0) and U is an upper-triangular matrix (all elements below the main diagonal are 0).
\[ \begin{split} \underset{n\times n}{\mathbf{A}} &= \underset{n\times n}{\mathbf{L}}~ \underset{n\times n}{\mathbf{U}} \\[2ex] &= \begin{bmatrix} l_{11} & 0 & 0 & \ldots & 0\\ l_{21}& l_{22} & 0 & \ldots & 0\\ l_{31}& l_{32} & l_{33} & \ldots & 0\\ \vdots & \vdots &\vdots & \ddots & \vdots \\ l_{n1} & l_{n2} & l_{n3} & \ldots & l_{nn} \end{bmatrix}\begin{bmatrix} u_{11} & u_{12} & u_{13} & \ldots & u_{1n}\\ 0 & u_{22} & u_{23} & \ldots & u_{2n}\\ 0 & 0 & u_{33} & \ldots & u_{3n}\\ \vdots & \vdots &\vdots & \ddots & \vdots \\ 0 & 0 & 0 & \ldots & u_{nn} \end{bmatrix} \end{split} \]
The goal of LU decomposition would be to find the values for each of the non-zero elements in L and U.
21.1 An Example of LU Decomposition
Consider the following \(2 \times 2\) matrix:
\[ \underset{2\times 2}{\mathbf{A}} = \begin{bmatrix} 5 & 1 \\ -4 & 2 \\ \end{bmatrix} \]
Then LU decomposition would define A as the product of a lower- and upper-triangular matrix:
\[ \begin{split} \underset{2\times 2}{\mathbf{A}} &= \underset{2\times 2}{\mathbf{L}}~\underset{2\times 2}{\mathbf{U}}\\[1em] \begin{bmatrix} 5 & 1 \\ -4 & 2 \\ \end{bmatrix} &= \begin{bmatrix} l_{11} & 0 \\ l_{21}& l_{22} \\ \end{bmatrix}\begin{bmatrix} u_{11} & u_{12} \\ 0 & u_{22} \\ \end{bmatrix} \end{split} \] To determine the elements of L and U, we can write out the system of equations based on the matrix algebra, we get:
\[ \begin{split} 5 &= l_{11}(u_{11}) + 0(0) \\ 1 &= l_{11}(u_{12}) + 0(u_{22})\\ -4 &= l_{21}(u_{11}) + l_{22}(0)\\ 2 &= l_{21}(u_{12}) + l_{22}(u_{22})\\ \end{split} \]
Unfortunately there are more unknowns (6) than equations (4) which means the system is underdetermined. To find a unique solution, we need to add additional constraints on the system. One way to do this is to require L to be a unit triangular matrix (i.e. elements on the main diagonal are ones). In our example, \(l_{11} = l_{22} = 1\), and our system of equations becomes:
\[ \begin{split} 5 &= 1(u_{11}) + 0(0) \\ 1 &= 1(u_{12}) + 0(u_{22})\\ -4 &= l_{21}(u_{11}) + 1(0)\\ 2 &= l_{21}(u_{12}) + 1(u_{22})\\ \end{split} \]
This system of equations is uniquely solvable (i.e., four equations; four unknowns). Solving for these unknowns, we find:
\[ \begin{split} l_{21} &= -0.8\\ u_{11} &= 5 \\ u_{12} &= 1\\ u_{22} &= 2.8\\ \end{split} \]
Thus, the LU decomposition of A is,
\[ \begin{bmatrix} 5 & 1 \\ -4 & 2 \\ \end{bmatrix} = \begin{bmatrix} 1 & 0 \\ -0.8 & 1 \\ \end{bmatrix}\begin{bmatrix} 5 & 1 \\ 0 & 2.8 \\ \end{bmatrix} \]
Checking our work in R, we find the solution holds.
# Create L
= matrix(
L data = c(1, -0.8, 0, 1),
nrow = 2
)
# Create U
= matrix(
U data = c(5, 0, 1, 2.8),
nrow = 2
)
# Product
%*% U L
[,1] [,2]
[1,] 5 1
[2,] -4 2
The determinant of the decomposed matrix (A) can be computed from finding the product of the diagonal elements of both the L and U matrices from the LU decomposition. From the example, the determinant of A is:
\[
\begin{split}
\mathrm{det}(\mathbf{A}) &= 1 \times 1 \times 5 \times 2.8 \\[2ex]
&= 14
\end{split}
\]
This is the same result as taking \(5(2) - (-4)(1) = 14\). The det()
function uses this method for computing the determinant of a matrix.
We can carry out LU decomposition using the lu.decomposition()
function from the matrixcalc package. This function outputs a list with the L and U matrices.
# Create A
= matrix(
A data = c(5, -4, 1, 2),
nrow = 2
)
# Load matrixcalc library
library(matrixcalc)
# PLU decomposition
= lu.decomposition(A)
lu_decomp
# View results
lu_decomp
$L
[,1] [,2]
[1,] 1.0 0
[2,] -0.8 1
$U
[,1] [,2]
[1,] 5 1.0
[2,] 0 2.8
Here the results are,
\[ \begin{split} {\mathbf{L}} &= \begin{bmatrix} 1 & 0 \\ -0.8 & 1 \\ \end{bmatrix} \\[1em] {\mathbf{U}} &= \begin{bmatrix} 5 & 1 \\ 0 & 2.8 \\ \end{bmatrix} \end{split} \]
To double-check that the decomposition worked, we can compute LU and see if we re-obtain A. We use the object name along with the $
notation to access each element of the output.
# Compute LU
$L %*% lu_decomp$U lu_decomp
[,1] [,2]
[1,] 5 1
[2,] -4 2
There are other methods of LU decomposition, including pivoting methods (PLU decomposition), which are more applicable in practice. There is also LDU decomposition in which A is decomposed into unit triangular matrices L and U, and diagonal matrix D.
21.2 Solving Systems of Equations with the LU Decomposition
Imagine if we wanted to solve a set of simultaneous equations to compute unknown values of B using our matrix A, and known values for Y, say,
\[ \mathbf{A}\mathbf{X}=\mathbf{Y} \]
To find the elements of X we would need the inverse of our matrix A. However, we know have an alternative solution from the LU decomposition. Since \(\mathbf{A}=\mathbf{L}\mathbf{U}\), we can re-write our simultaneuous equations as:
\[ \mathbf{L}\mathbf{U}\mathbf{X}=\mathbf{Y} \]
Pre-multiplying this by L inverse, we get
\[ \begin{split} \mathbf{L}^{-1}\mathbf{L}\mathbf{U}\mathbf{X}&=\mathbf{L}^{-1}\mathbf{Y} \\[0.5em] \mathbf{U}\mathbf{X}&=\mathbf{L}^{-1}\mathbf{Y} \end{split} \]
Let us call the right-hand side of this equation Z. This gives us two sets of equations related to Z:
\[ \begin{split} \mathbf{U}\mathbf{X} &= \mathbf{Z} \\[0.5em] \mathbf{L}^{-1}\mathbf{Y} &= \mathbf{Z} \end{split} \]
The second equation, we can also express (after pre-multiplying by L) as \(\mathbf{L}\mathbf{Z} = \mathbf{Y}\). Thus we now have the two equations that are now based on the matrices L and U from our decomposition.
\[ \begin{split} \mathbf{U}\mathbf{X} &= \mathbf{Z} \\[0.5em] \mathbf{L}\mathbf{Z} &= \mathbf{Y} \end{split} \]
Remember, the goal was to solve for X, so to do this, we first solve the second equation, \(\mathbf{L}\mathbf{Z} = \mathbf{Y}\), for Z (which is unknown), and then use that to solve the first equation for X. Let’s see it in action using our example. To do so, let’s solve this set of simultaneous equations:
\[ \underset{\mathbf{A}}{\begin{bmatrix} 5 & 1 \\ -4 & 2 \\ \end{bmatrix}}\underset{\mathbf{X}}{\begin{bmatrix} x_{11} \\ x_{21} \\ \end{bmatrix}} = \underset{\mathbf{Y}}{\begin{bmatrix} 1 \\ -3 \\ \end{bmatrix}} \]
21.2.1 Step 1: Solve for Z
\[ \begin{split} \mathbf{L}\mathbf{Z} &= \mathbf{Y} \\[0.5em] \begin{bmatrix} 1 & 0 \\ -0.8 & 1 \\ \end{bmatrix}\begin{bmatrix} z_{11} \\ z_{21} \\ \end{bmatrix} &= \begin{bmatrix} 1 \\ -3 \\ \end{bmatrix} \end{split} \] The two equations from this multiplication are:
\[ \begin{split} z_{11} &= 1 \\[0.5em] -0.8z_{11} + z_{21} &= -3 \end{split} \]
The triangular matrix makes this really easy to solve these equations for the two elements of Z. Since solving these equations boils down to substituting values into each subsequent equation, this method of solving the equations is referred to as forward substitution. Here,
\[ \begin{split} z_{11}&=1 \\[2ex] z_{21}&=-2.2 \end{split} \]
We can now use these values of Z in the second equation.
21.2.2 Step 2: Solve for X
\[ \begin{split} \mathbf{U}\mathbf{X} &= \mathbf{Z} \\[0.5em] \begin{bmatrix} 5 & 1 \\ 0 & 2.8 \\ \end{bmatrix} \begin{bmatrix} x_{11} \\ x_{21} \\ \end{bmatrix} &= \begin{bmatrix} 1 \\ -2.2 \\ \end{bmatrix} \end{split} \]
The two equations from this multiplication are:
\[ \begin{split} 5(x_{11}) + x_{21} &= 1 \\[0.5em] 2.8(x_{21}) &= -2.2 \end{split} \]
Again, the triangular matrix makes this really easy to solve these equations for the two elements of X. Solving these equations boils down to substituting values from later equations into each of the earlier equations. Hence, this method of solving the equations is referred to as back substitution. After carrying out the algebra,
\[ \mathbf{B} \approx\begin{bmatrix} 0.36 \\ -0.79 \\ \end{bmatrix} \]
The exciting thing here is that we have solved for X without ever finding the inverse of the A matrix! It turns out that this is also a much more computationally efficient method to solve systems of equations. (Even though it maybe didn’t feel like it when we used a \(2 \times 2\) matrix.)
21.2.3 Using R to Solve the Two Equations
We can also use R to solve the two equations, \(\mathbf{LZ}=\mathbf{Y}\) and \(\mathbf{UX}=\mathbf{Z}\). The function forwardsolve()
(forward substitution) can be used to solve the equation \(\mathbf{LZ}=\mathbf{Y}\), and more generally, any equation where a lower-triangular matrix is being pre-multiplied by a matrix of unknown elements to produce another known matrix. This function takes a lower-triangular matrix as its first argument and the solution matrix as its second argument. The syntax to solve the following equation for the elements of Z is given below.
\[ \begin{split} \mathbf{L}\mathbf{Z} &= \mathbf{Y} \\[0.5em] \begin{bmatrix} 1 & 0 \\ -0.8 & 1 \\ \end{bmatrix}\begin{bmatrix} z_{11} \\ z_{21} \\ \end{bmatrix} &= \begin{bmatrix} 1 \\ -3 \\ \end{bmatrix} \end{split} \]
# Create L
= matrix(
L data = c(1, -0.8, 0, 1),
nrow = 2
)
# Create Y
= matrix(
Y data = c(1, -3),
nrow = 2
)
# Solve for Z
= forwardsolve(L, Y)
Z
# View Z
Z
[,1]
[1,] 1.0
[2,] -2.2
To solve an upper-triangular system of equations—one in which an upper-triangular matrix is being pre-multiplied by a matrix of unknown elements to produce another known matrix—we use the backsolve()
function (backward substitution). This function takes an upper-triangular matrix as its first argument and the solution matrix as its second argument. The syntax to solve the following equation for the elements of X is given below.
\[ \begin{split} \mathbf{U}\mathbf{X} &= \mathbf{Z} \\[0.5em] \begin{bmatrix} 5 & 1 \\ 0 & 2.8 \\ \end{bmatrix} \begin{bmatrix} x_{11} \\ x_{21} \\ \end{bmatrix} &= \begin{bmatrix} 1 \\ -2.2 \\ \end{bmatrix} \end{split} \]
# Create L
= matrix(
U data = c(5, 0, 1, 2.8),
nrow = 2
)
# Solve for X
= backsolve(U, Z)
X
# View X
X
[,1]
[1,] 0.3571429
[2,] -0.7857143
These functions can also take as arguments the lower- or upper-triangular matrices produced from the lu()
function. For example:
# Solve for Z
= forwardsolve(lu_decomp$L, Y)
Z
# Solve for X
= backsolve(lu_decomp$U, Z)
X
# View X
X
[,1]
[1,] 0.3571429
[2,] -0.7857143
21.3 Application of LU Decomposition in Computing
One application of LU decomposition in computing is in the computation of a determinant. The determinant is often computed by taking the product of the elements on the diagonal of both the L and U matrices. Since LU decomposition is quite efficient, this is a computationally efficient way of computing the determinant. The det()
function R, for example, uses this method to compute the determinant. This method, however, can lead to “wrong” results, especially for matrices that have a determinant of 0.
Consider the following matrix,
\[ \mathbf{A} = \begin{bmatrix} 3 & 2 \\ 1.2 & 0.8 \\ \end{bmatrix} \]
The determinant can be computed as:
\[ \begin{split} \mathrm{det}(\mathbf{A}) &= 3(0.8) - 1.2(2) \\ &= 2.4 - 2.4 \\ &= 0 \end{split} \]
However, if we use the det()
function on the matrix, we find:
# Create A
= matrix(
A data = c(3, 1.2, 2, 0.8),
nrow = 2
)
# Compute determinant
det(A)
[1] 3.330669e-16
While close to 0, the det()
function does not return 0.
This function, as mentioned earlier, computes the determinant by carrying out an LU decomposition using a Fortran routine called LAPACK (Linear Algebra PACKage). This routine is also used in the lu.decomposition()
function.
# Use LAPACK routine for LU decomposition
lu.decomposition(A)
$L
[,1] [,2]
[1,] 1.0 0
[2,] 0.4 1
$U
[,1] [,2]
[1,] 3 2.000000e+00
[2,] 0 1.110223e-16
Note that the diagonal elements of these matrices are 1, 1, 3, and \(1.110223\times 10^{-16}\). Multiplying these together, we get \(3.330669\times 10^{-16}\), the same result as we got from det()
. The routines used to carry out the LU decomposition are computationally approximate, and suffer from the same floating point issues that all computation suffers from. Because of this, the U matrix has a diagonal element of \(1.110223\times 10^{-16}\) rather than 0.
Note that using zero in the U matrix produces the correct decomposition.
\[ \begin{split} \underset{\mathbf{A}}{\begin{bmatrix} 3 & 2 \\ 1.2 & 0.8 \\ \end{bmatrix}} &= \underset{\mathbf{L}}{\begin{bmatrix} 1 & 0 \\ 0.4 & 1 \\ \end{bmatrix}}\underset{\mathbf{U}}{\begin{bmatrix} 3 & 2 \\ 0 & 0 \\ \end{bmatrix}}\\[1em] \begin{bmatrix} 5 & 1 \\ -4 & 2 \\ \end{bmatrix} &= \begin{bmatrix} l_{11} & 0 \\ l_{21}& l_{22} \\ \end{bmatrix}\begin{bmatrix} u_{11} & u_{12} \\ 0 & u_{22} \\ \end{bmatrix} \end{split} \]